Lead Organisation: University College London, Mullard Space Science Laboratory (MSSL)
Project Lead: Prof. Jan Peter Muller
Partners: University College London (UCL) & Surrey Satellite Technology Limited (SSTL)
Very high spatial resolution imaging data is playing an increasingly important role in many commercial and scientific applications of Earth observation. However, given the physical constraints of the imaging instruments themselves, we always need to trade-off spatial resolution against launch mass, usable swath-width, and telecommunications bandwidth for transmitting data back to Earth. One solution to this conundrum is through the use of super-resolution restoration (SRR) processing to combine image information from repeat observations at multiple viewing angles, and exploit information derived from multiple imaging sources using deep learning, to generate images at much higher spatial resolutions.
In the CEOI 10 SuperRes-EO project, a novel SRR system called MAGiGAN was developed and demonstrated to improve native image resolution by 3-5 times using multi-pass Earth observation (EO) images or video sequences. MAGiGAN stands for Multi-Angle Gotcha image restoration with Generative Adversarial Network. The MAGiGAN SRR system uses a combination of photogrammetric and machine vision approaches including image segmentation and shadow labelling, feature matching and densification, estimation of an image degradation model, and deep learning approaches. The MAGiGAN SRR system not only retrieves subpixel information from multi-angle distorted features, but also uses the deep learning GAN network to retrieve high-frequency texture details in low texture regions.
SRR can be performed either as post processing on the Earth or potentially via satellite onboard processing using a graphics processing unit (GPU). In SuperRes-EO, the MAGiGAN SRR system was implemented and tested on a lower power GPU card (NVIDIA Jetson TX-2) and achieved speed-ups for the majority of the algorithm of up to 20 times when compared with a large multi-core Linux workstation. This has huge potential for onboard processing within a smart-satellite capturing high definition satellite videos, which will enable many innovative remote-sensing applications to be developed in the future.
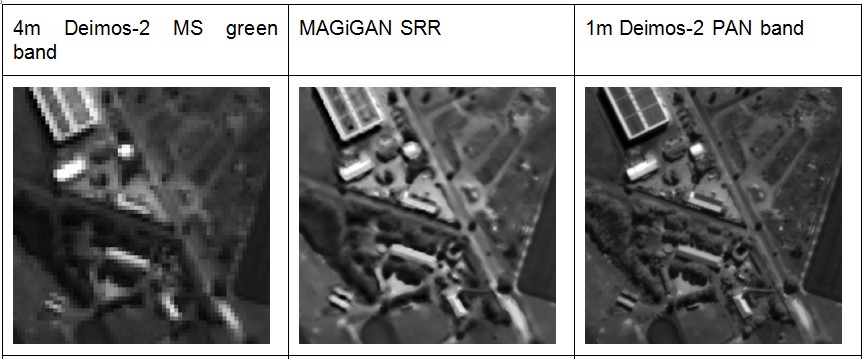
In SuperRes-EO, the MAGiGAN SRR system was applied to stacks of 4m UrtheCast Corp Deimos-2 (green band) multi-angle repeat-pass images over several experimental sites to produce SRR results with 3.5–3.75 times resolution enhancement (see Figure below). It was also tested with 1.1m SSTL Carbonite-2 video frames and subsequently with 70cm Planet® Skysat® HD 70cm videos, 300m Sentinel 3 OLCI level-1 EFR red band radiance images and 275m Multi-angle Imaging SpectroRadiometer (MISR) Level 1B1 red band images to produce resolution enhancement at 3-4 times.