Lead Organisation: Craft Prospect Ltd
Project Lead: Steve Greenland
Partners: University College London, University of Manchester and Bright Ascension Ltd.
Progress within nanosatellite systems development makes niche EO missions feasible. However these systems will remain downlink limited, so it is important to ensure that data sent down has maximum data content. The embedding of existing ground-based image processing algorithms into onboard systems is non-trivial especially in limited resource nanosatellites, necessitating new approaches. Craft Prospect led a consortium investigating emerging technologies and techniques for enabling onboard data autonomy targeted for the low resources available on nanosatellites. The work surveyed existing missions and techniques, and looked out of sector including algorithms used in autonomous vehicles and commercial machine learning.
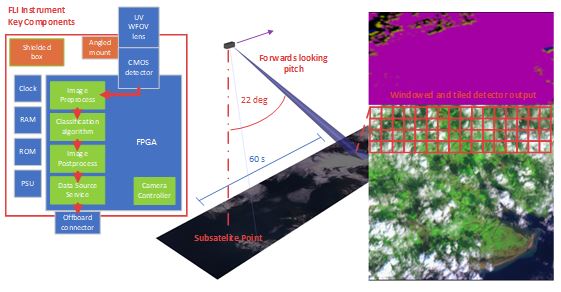
As an exemplar, the overall autonomy framework for Earth observation nanosatellites was developed as a miniaturised camera system with FPGA-based deep learning neural network capabilities. For proof of concept, the system has been trained for real time cloud detection and classification, looking 1 min ahead of the satellite to enable responsive decision making for Earth observation and telecommunication applications. The design has been miniaturised and modularised to allow accommodation on small and nanosatellite systems. Flight representative and heritage components have been selected for prototyping. A simulator to facilitate end to end testing of the system has been developed using existing data sets as input, incorporating distortions to test robustness. Results show that a competitive low power < 2 W system can be delivered, with the chain response < 5 seconds from data capture to input into the onboard planning and with timing consistent with continuous real time decision-making in Low Earth Orbit.